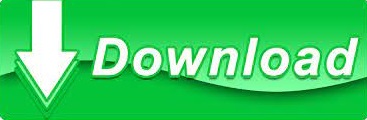
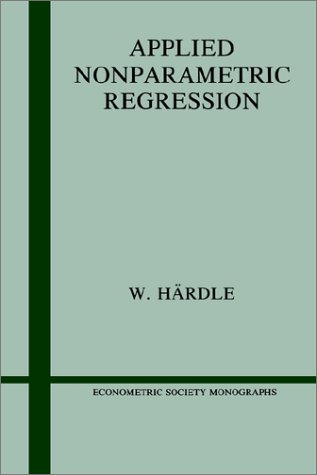

Even a simple 5-number summary can expose more useful information than more traditional methods by exposing skew and other useful underlying features. I can say that on real-world non-experimental datasets I find nonparametric methods far more useful than trying to fit to a theoretical distribution. You might also consider one of the books by Good relating to permutation tests (and other resampling procedures)

Some (old) references would be Conover's Applied nonparametric statistics and the book by Daniel (whose title escapes me right now, but is reasonably comprehensive). On light tailed distributions, it often leans the other way.Ģ) What are some good books/resources for the field?ĭepends on what you want, and at what level. If you're considering whether to do a t-test against a Wilcoxon-Mann-Whitney for a location shift with heavy tailed near-symmetric distributions, there's often a clear advantage for the nonparametric one. The computer's really useful for permutation/randomization tests.ĭoes it have advantages over parametric stats for very large datasets? Yes and no it also makes alternatives, like robust statistics, more feasible.Īs a result it depends somewhat on the application area - some areas are using them more, others less, some about the same. With the advent of statistical computing, has nonparametric statistics gained more prominence? You could even have both at the same time. One is where a parametric distributional form is not assumed ("distribution-free") and the other is where a parametric form of relationship is not assumed (such as "nonparametric regression").

There's actually two things to which 'nonparametric' is applied both relate to weaker assumptions.
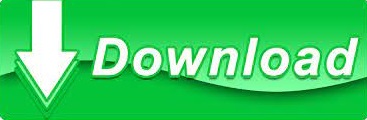